In this study, the performance and hydrological utility of IMERG rainfall estimates over the Upper Bhima River basin, India, are comprehensively evaluated using a VIC-RAPID hydrologic model. Moreover, a bias-correction scheme based on the long short-term memory (LSTM) neural network method is proposed, and the results are compared with two commonly used bias-correction techniques. Results indicated that the spatial distribution of observed rainfall is well captured by IMERG; however, it showed a general tendency of overestimation, especially on a daily timescale. The LSTM-based approach showed notable improvements against the other bias-correction techniques substantiating its utility for accurately estimating rainfall amount and skillful detection of rainfall events in the study area. The VIC-RAPID model simulations for hydrological variables revealed a significant improvement in the performance of the bias-corrected IMERG product. In addition, the effect of the hydrological model recalibration with the different rainfall input datasets has also been elucidated.
Hydrological utility of LSTM-corrected IMERG
Evaluating the performance of bias-corrected IMERG satellite rainfall estimates for hydrological simulation over the Upper Bhima River basin, India
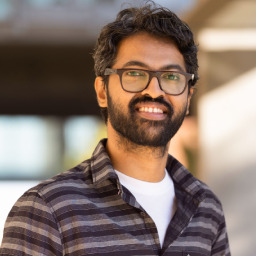
WRITTEN BY
Saswata Nandi
Postdoc@SNRI, UC Merced